Leverage to的問題,透過圖書和論文來找解法和答案更準確安心。 我們找到下列問答集和懶人包總整理
Leverage to的問題,我們搜遍了碩博士論文和台灣出版的書籍,推薦寫的 Preparing for Marriage: 5 Conversations to Have Between the Engagement Ring and the Wedding Ring 和Vacca, John R.的 Computer Forensics: Computer Crime Scene Investigation都 可以從中找到所需的評價。
這兩本書分別來自 和所出版 。
國立陽明交通大學 資訊科學與工程研究所 謝秉均所指導 謝秉瑾的 貝氏最佳化的小樣本採集函數學習 (2021),提出Leverage to關鍵因素是什麼,來自於貝氏最佳化、強化學習、少樣本學習、機器學習、超參數最佳化。
而第二篇論文國立陽明交通大學 資訊科學與工程研究所 吳育松所指導 鮑俊安的 基於記憶體存取事件取樣觀測及低耦合汙染源追蹤之記憶體資訊流追蹤技術 (2021),提出因為有 虛擬機管理器、資訊流、動態汙染分析、記憶體監測、可疑行為偵測、變數識別化技術的重點而找出了 Leverage to的解答。
Preparing for Marriage: 5 Conversations to Have Between the Engagement Ring and the Wedding Ring
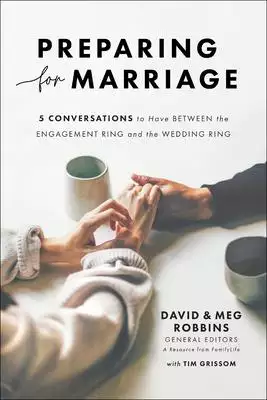
為了解決Leverage to 的問題,作者 這樣論述:
David Robbins became president of FamilyLife in December 2017. He and his wife, Meg, desire to leverage FamilyLife’s resources and build upon the over four-decade foundation of the organization while looking to reach new audiences and generations to come. Prior to David’s role with FamilyLife, the R
obbinses lived in Manhattan and David served as a national co-facilitator for Cru’s millennials ministry. He, Meg, and their four children currently reside in Orlando, Florida.
Leverage to進入發燒排行的影片
Learn how to leverage FLink to share private, draft, and scheduled episodes to your production team or exclusive-content community!
BRIDGE THE GAP between podcasters and audiences. Create, distribute, grow, and monetize your show on Firstory!
[Get started for free:] https://firstory.me/
[Follow us on Twitter:] https://twitter.com/firstorylab
貝氏最佳化的小樣本採集函數學習
為了解決Leverage to 的問題,作者謝秉瑾 這樣論述:
貝氏最佳化 (Bayesian optimization, BO) 通常依賴於手工製作的採集函數 (acqui- sition function, AF) 來決定採集樣本點順序。然而已經廣泛觀察到,在不同類型的黑 盒函數 (black-box function) 下,在後悔 (regret) 方面表現最好的採集函數可能會有很 大差異。 設計一種能夠在各種黑盒函數中獲得最佳性能的採集函數仍然是一個挑戰。 本文目標在通過強化學習與少樣本學習來製作採集函數(few-shot acquisition function, FSAF)來應對這一挑戰。 具體來說,我們首先將採集函數的概念與 Q 函數 (Q
-function) 聯繫起來,並將深度 Q 網路 (DQN) 視為採集函數。 雖然將 DQN 和現有的小樣本 學習方法相結合是一個自然的想法,但我們發現這種直接組合由於嚴重的過度擬合(overfitting) 而表現不佳,這在 BO 中尤其重要,因為我們需要一個通用的採樣策略。 為了解決這個問題,我們提出了一個 DQN 的貝氏變體,它具有以下三個特徵: (i) 它 基於 Kullback-Leibler 正則化 (Kullback-Leibler regularization) 框架學習 Q 網絡的分佈(distribution) 作為採集函數這本質上提供了 BO 採樣所需的不確定性並減輕了
過度擬 合。 (ii) 對於貝氏 DQN 的先驗 (prior),我們使用由現有被廣泛使用的採集函數誘導 學習的演示策略 (demonstration policy),以獲得更好的訓練穩定性。 (iii) 在元 (meta) 級別,我們利用貝氏模型不可知元學習 (Bayesian model-agnostic meta-learning) 的元 損失 (meta loss) 作為 FSAF 的損失函數 (loss function)。 此外,通過適當設計 Q 網 路,FSAF 是通用的,因為它與輸入域的維度 (input dimension) 和基數 (cardinality) 無 關。通過廣
泛的實驗,我們驗證 FSAF 在各種合成和現實世界的測試函數上實現了與 最先進的基準相當或更好的表現。
Computer Forensics: Computer Crime Scene Investigation
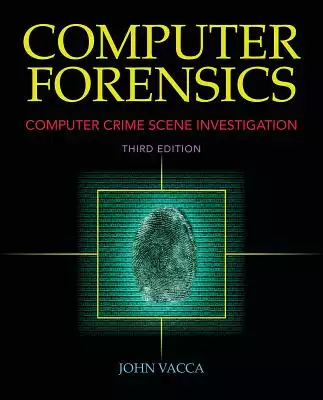
為了解決Leverage to 的問題,作者Vacca, John R. 這樣論述:
Despite efforts to safeguard sensitive data and networks, organizations face an ever-growing threat of cyber crime and security violations. These attacks can occur internally as well as from an external source, and include fraud, copyright infringement, and stolen data. This computer forensics and i
ncident response book provides an organization's internal computer security specialists with a legal method for handling computer misuse as well as a means for securing sensitive data and identifying compromised systems, ultimately saving the organization the high cost of hiring an outside team of c
omputer forensic investigators. This updated Third Edition includes a comprehensive introduction to computer forensics investigative techniques and provides the knowledge and skills required to conduct a computer forensics investigation from initial discovery to completion. This book also provides t
he details for an organization's Computer Incident Response Team (CIRT); to collect, manage, and record digital evidence; and to leverage powerful software tools and techniques to uncover hidden or deleted information. Key topics include: Responding to incidents and investigating computer crime, con
ducting and managing an investigation, performing disk-based analysis, investigating information-hiding techniques, examining e-mail, tracing internet access, searching memory in real-time, and the forensics challenge competition.
基於記憶體存取事件取樣觀測及低耦合汙染源追蹤之記憶體資訊流追蹤技術
為了解決Leverage to 的問題,作者鮑俊安 這樣論述:
資訊流追蹤已被發展多年,此種技術可被用來偵測目標程式之非正常行為,例如外部輸入對程式之影響、機敏資料洩漏、緩衝區覆寫攻擊等等。在過去的研究中,多數選擇使用插入特定程式碼以監控資訊流動,往往造成很大的系統負擔導致效能低落。我們提出在程式執行時期進行系統層級記憶體狀態採樣,並且非同步進行汙染追蹤模擬的方式,以達到同時滿足效能及準確度的目的。根據我們的實驗,在Nginx中只造成約1.6%的效能負擔,在單元測試中有約93%的結果與Taintgrind之結果相符。同時,我們加入變數識別化系統及資訊流視覺化系統,使實驗結果能更清楚呈現。