Be leveraged to的問題,透過圖書和論文來找解法和答案更準確安心。 我們找到下列問答集和懶人包總整理
Be leveraged to的問題,我們搜遍了碩博士論文和台灣出版的書籍,推薦Kiernan, Vincent寫的 Atomic Bill: A Journalist’’s Dangerous Ambition in the Shadow of the Bomb 和Feld, Brad,Ramsinghani, Mahendra,Blumberg, Matt的 Startup Boards: Getting the Most Out of Your Board of Directors都 可以從中找到所需的評價。
這兩本書分別來自 和所出版 。
國立陽明交通大學 財務金融研究所 戴天時所指導 鄧名勛的 使用等級相依期望效用模型與資產森林結構推論實證之員工認股權發行決策 (2021),提出Be leveraged to關鍵因素是什麼,來自於員工認股選擇權、資產森林評價、等級相依期望效用、機率加權函數、部分履約。
而第二篇論文東吳大學 會計學系 高立翰所指導 馮少辰的 以機器學習方式辨認財務危機公司 -納入重大訊息之考量 (2021),提出因為有 財務危機、重大訊息、機器學習、文字探勘的重點而找出了 Be leveraged to的解答。
Atomic Bill: A Journalist’’s Dangerous Ambition in the Shadow of the Bomb
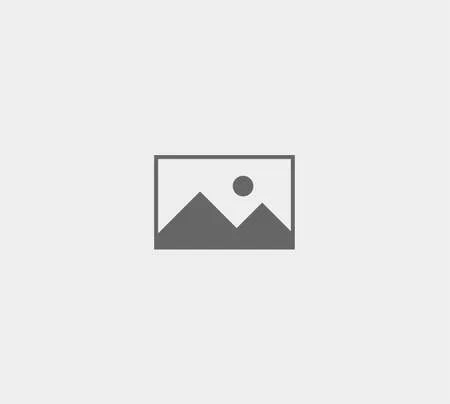
為了解決Be leveraged to 的問題,作者Kiernan, Vincent 這樣論述:
In Atomic Bill, Vincent Kiernan examines the fraught career of New York Times science journalist, William L. Laurence and shows his professional and personal lives to be a cautionary tale of dangerous proximity to power. Laurence was fascinated with atomic science and its militarization. When the Ma
nhattan Project drew near to perfecting the atomic bomb, he was recruited to write much of the government’s press materials that were distributed on the day that Hiroshima was obliterated. That instantly crowned Laurence as one of the leading journalistic experts on the atomic bomb. As the Cold War
dawned, some assessed Laurence as a propagandist defending the militarization of atomic energy. For others, he was a skilled science communicator who provided the public with a deep understanding of the atomic bomb. Laurence leveraged his perch at the Times to engage in paid speechmaking, book writi
ng, filmmaking, and radio broadcasting. His work for the Times declined in quality even as his relationships with people in power grew closer and more lucrative. Atomic Bill reveals extraordinary ethical lapses by Laurence such as a cheating scandal at Harvard University and plagiarizing from press
releases about atomic bomb tests in the Pacific. In 1963 a conflict of interest related to the 1964 World’s Fair in New York City led to his forced retirement from the Times. Kiernan shows Laurence to have set the trend, common among today’s journalists of science and technology, to prioritize gee-w
hiz coverage of discoveries. That approach, in which Laurence served the interests of governmental official and scientists, recommends a full revision of our understanding of the dawn of the atomic era. Vincent Kiernan is the Dean of the Metropolitan School of Professional Studies at The Catholic
University of America in Washington DC. He is the author of Embargoed Science, Writing Your Dissertation with Microsoft Word, and Finding an Online High School.
Be leveraged to進入發燒排行的影片
✅ Daftar Akun eToro : https://linktr.ee/sahamworld
-------------------------------------------------
Disclaimers:
eToro AUS Capital Pty Ltd, ABN 66 612 791 803 AFSL 491139. CFDs are highly leveraged and risky, and may not be suitable for all investors. You may lose more than your initial investment. Trading CFDs does not result in ownership of the underlying assets. You should obtain your own advice and refer to our FSG and PDS before deciding whether to trade with us.
使用等級相依期望效用模型與資產森林結構推論實證之員工認股權發行決策
為了解決Be leveraged to 的問題,作者鄧名勛 這樣論述:
員工認股選擇權 (ESO)是常見的權益連結型薪酬,我們建構了全面的資產森林評價 模型,以一名代表員工在等級相依期望效用模型 (RDEU)下進行最佳化決策。我們的模 型考慮了員工的跨期選擇、部分履約,以及不同種類的認股權 (分為 NQSO和 QSO)在 稅制上的差異,也考慮 ESO履約時的注資、稀釋效果 (還有 NQSO的稅盾效果 ),並加入機率加權函數,讓員工存在決策機率偏誤。有別過往文獻以 ESO確定等值 (CE)和成本的比較,我們觀察四種不同最佳決策下, ESO占最適薪酬組合的比例。在我們模型中的ESO可處理履約對資產結構的改變,並發現機率加權函數會對ESO評價有明顯的影響,且在「固定員
工感受度,極大化公司資產價值」最佳化下,能夠普遍解釋ESO在實證上觀察到的現象,諸如「市面上NQSO多於QSO」、「波動度越大的公司其發行ESO量越多」、「部分CEO拿接近零現金的薪酬組合」以及「員工部分履約」的現象。因此我們推論此最佳化可能是大多數的公司在訂定員工薪酬計畫時的主要策略。
Startup Boards: Getting the Most Out of Your Board of Directors
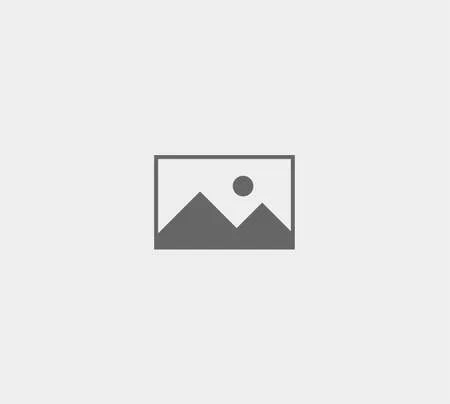
為了解決Be leveraged to 的問題,作者Feld, Brad,Ramsinghani, Mahendra,Blumberg, Matt 這樣論述:
A comprehensive guide on creating, growing, and leveraging a board of directors written for CEOs, board members, and people seeking board roles. The first time many founders see the inside of a board room is when they step in to lead their board. But how do boards work? How should they be structu
red, managed, and leveraged so that startups can grow, avoid pitfalls, and get the best out of their boards? Authors Brad Feld, Mahendra Ramsinghani, and Matt Blumberg have collectively served on hundreds of startup and scaleup boards over the past 30 years, attended thousands of board meetings, enc
ountered multiple personalities and situations, and seen the good, bad, and ugly of boards. In Startup Boards: A Field Guide to Building and Leading an Effective Board of Directors, the authors provide seasoned advice and guidance to CEOs, board members, investors, and anyone aspiring to serve on a
board. This comprehensive book covers a wide range of topics with relevant tips, tactics, and best practices, including: Board fundamentals such as the board’s purpose, legal characteristics, and roles and functions of board members;Creating a board including size, composition, roles of VCs and inde
pendent directors, what to look for in a director, and how to recruit directors;Compensating, onboarding, removing directors, and suggestions on building a diverse board;Preparing for and running board meetings;The board’s role in transactions including selling a company, buying a company, going pub
lic, and going out of business;Advice for independent and aspiring directors.Startup Boards draws on the authors’ experience and includes stories from board members, startup founders, executives, and investors. Any CEO, board member, investor, or executive interested in creating an active, involved,
and engaged board should read this book--and keep it handy for reference.
以機器學習方式辨認財務危機公司 -納入重大訊息之考量
為了解決Be leveraged to 的問題,作者馮少辰 這樣論述:
過去研究所建立的財務危機預測模型大抵分為兩類,一為利用財務指標從公司的財務面找出公司破產的徵兆,二為加入非財務方面資訊,如公司治理相關指標,結合財務面以加強對於公司財務危機預測的準確率。近期受惠於網路發達,大量數位化文字資料的產生,也提高了文字資訊對於判斷財務危機時的重要性及影響。有鑑於此,本研究欲以公開資訊觀測站中之重大訊息納入財務危機預測模型,以提供不同利害關係人更多面向的考量。實證結果顯示,當公司發布重大訊息含有特定關鍵詞,且其中負面詞彙出現頻率越高,則公司越容易存有財務危機。而重大訊息中之中性詞彙則可以作為排除與財務危機無關之訊息之參考,以縮小存有可能產生財務危機訊息的範圍。最後,本
研究使用共四種機器學習方法來訓練並驗證納入重大訊息關鍵詞後之財務危機預警模型準確度。辨認方法準確率由高至低分別為隨機森林73.35%、羅吉斯迴歸65.96%、支援向量機63.72及K-近鄰演算法55.15,最終結果可得到隨機森林法為最佳預測模型。